Startup
Traditional vs. AI-Powered Search Engines: Navigating the Future of Search
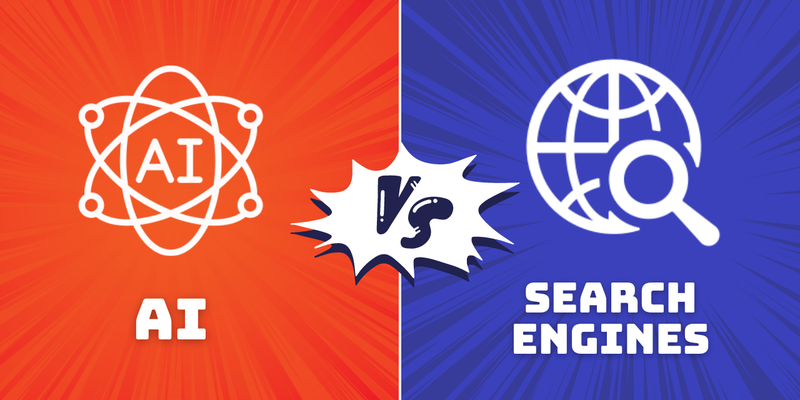
The rise of AI has shifted the landscape of search engines, bringing forward an exciting array of possibilities. But how do these AI-powered search engines differ from the classic, keyword-driven engines like Google and Bing? Below, we break down the pros and cons of each, backed by data insights, to give a clear view of their strengths and limitations.
Traditional search engines rely on algorithms that rank pages based on keywords, backlinks, and website authority. They are designed for speed and comprehensive coverage, making them the go-to option for straightforward queries.
Pros of Traditional Search Engines
Speed and Scalability:
- With over 2 trillion searches annually on Google alone, traditional engines are optimised to handle massive traffic and deliver results in fractions of a second. Google processes about 63,000 searches per second, demonstrating unmatched scalability.
- Data shows users expect search engines to load results within 1 second, with a delay leading to 11% fewer searches.
Breadth of Information:
- Traditional engines index a massive amount of data. For example, Google has indexed over 50 billion web pages, ensuring vast coverage. This ensures users receive a comprehensive range of sources across various domains.
- Their data-indexing capability covers almost 90% of searchable web content, offering reliable access to public information.
Refined Search Ranking System:
- Traditional search engines rely on hundreds of ranking factors, with studies suggesting that backlinks, keywords, and on-page optimisation account for around 50% of ranking weightage.
- This allows users to access results that prioritise high-authority, well-regarded sources, enhancing content reliability.
Cons of Traditional Search Engines
Keyword Sensitivity:
- The reliance on exact keyword matching often results in irrelevant links if queries aren’t specific enough. In fact, 35% of searches require keyword adjustments for better results, highlighting the limitations in handling nuanced or ambiguous searches.
- This keyword dependency limits the ability to answer conversational or complex queries effectively.
Inability to Interpret Context:
- Search engines lack advanced contextual understanding, leading to mismatches in search intent for queries that aren’t straightforward.
- About 40% of users reportedly struggle with search engines not interpreting their intent effectively on the first attempt, according to Google’s own data.
Information Overload:
- Users often face a flood of links, with 75% of users never clicking past the first page. This setup can lead to surface-level information retrieval, leaving users with an overwhelming number of choices without deeper answers.
AI-powered search engines use natural language processing (NLP) and machine learning models to understand user intent better, aiming to bridge the gap between simple keyword matching and human-like comprehension.
Pros of AI Search Engines
Enhanced Contextual Understanding:
- AI search engines can discern nuanced queries and deliver tailored responses. For instance, 76% of users report they prefer AI-enhanced engines for handling complex questions.
- NLP allows these engines to capture intent and context, making them adept at processing ambiguous queries. This has led to an 18% higher user satisfaction in complex search scenarios.
Personalised Search Experience:
- AI search engines analyse user data to tailor responses, with around 72% of users prefer personalised search results that adapt to their preferences and history.
- Google’s recent advancements in AI, for example, have shown a 25% increase in personalised results accuracy due to adaptive learning.
Conversational Interaction:
- Many AI-powered engines enable follow-up queries, facilitating a conversational experience. Chat-based AI search engines, like ChatGPT with its browsing feature, allow users to refine searches iteratively.
- This dynamic interaction has shown an 87% increase in user engagement, especially among Gen Z and millennials.
Cons of AI Search Engines
Privacy Concerns and Data Usage:
- The need for personalisation brings data privacy into question. Experts indicate that 80% of users express concerns about the amount of data AI models require for personalised results.
- GDPR compliance and user data consent remain challenges, with only 43% of AI search engine companies fully transparent about data usage.
Computational Resource Demand:
- AI models are resource-intensive, consuming up to 10 times the computational power of traditional search algorithms. OpenAI’s GPT-3, for instance, used around 700,000 liters of water for cooling per day during peak training phases.
- The environmental impact and high operating costs make scalability and accessibility challenging, especially for smaller companies.
Algorithmic Bias:
- AI engines can perpetuate biases present in their training data, leading to skewed or controversial results. Around 58% of users have noticed biases in AI-generated responses, with researchers actively working to mitigate this.
GPT Search represents a cutting-edge approach to AI-driven search. Leveraging the GPT-4 model, it offers capabilities that redefine the search experience by providing real-time answers, interactive source links, and conversational flexibility.
How GPT Search Stands Out
Real-Time Information Access:
- GPT Search, with its browsing functionality, can fetch up-to-date information, offering users the latest content. This capability ensures more relevance, especially for time-sensitive queries, and positions it as a powerful research tool.
Interactive Source Linking:
- One of GPT Search’s innovations is allowing users to click on source links directly within the chat interface. This feature supports transparency and lets users verify information on the go, a key demand among 68% of users who prioritise source credibility.
Contextual, In-Depth Answers:
- GPT Search can maintain context across multiple queries, allowing users to delve deeper into topics through conversational prompts. This leads to an 82% satisfaction rate for users exploring multi-faceted topics or looking for detailed explanations.
Limitations of GPT Search
Accuracy and Hallucination Issues:
- Despite its advancements, GPT Search can sometimes produce inaccurate or “hallucinated” responses, where it fabricates plausible but incorrect answers. According to OpenAI, approximately 15% of complex responses require user verification to ensure accuracy.
- This limitation underscores the need for careful verification, particularly for professional use cases.
Cost and Accessibility:
- Running models like GPT-4 incurs substantial costs, potentially impacting accessibility for broader audiences. With AI-driven costs being 6 to 7 times higher than traditional engines, affordability remains a challenge.
- These costs also influence availability, with free versions often limited in features compared to premium counterparts.
Legal and Ethical Concerns:
- Using public content for AI training raises issues around copyright and fair use, with regulations in this area still evolving. Studies suggest that 65% of legal experts foresee increased AI-related litigation over content usage and intellectual property rights.
Both traditional and AI-powered search engines have distinct strengths and areas for improvement. Traditional search engines deliver speed, extensive indexing, and familiarity, making them excellent for straightforward queries. AI search engines, with advanced contextual understanding and personalisation, offer a more intuitive experience for complex queries, albeit with privacy, cost, and accuracy challenges.
GPT Search stands as a promising evolution, blending the best of AI-powered capabilities with conversational depth, yet it grapples with accuracy and accessibility concerns. As search technology advances, striking a balance between these benefits and limitations will define the next wave of innovations in digital search.
Startup
Freshworks reports 22% jump in revenue to $186.6M in Q3
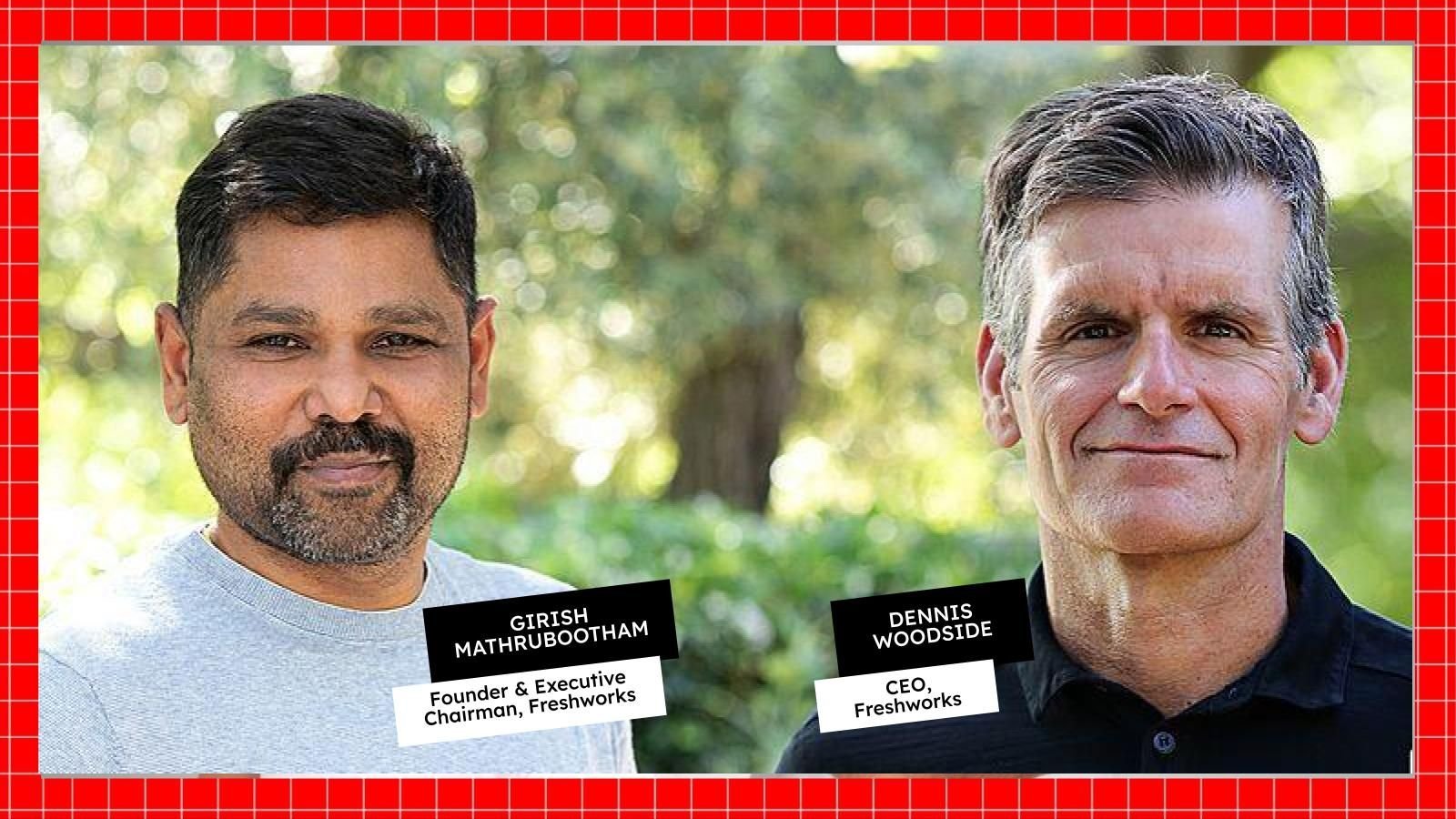
Software-as-a-Service (SaaS) major
has reported a 22% growth in revenue, climbing to $186.6 million for the third quarter ended September 30, 2024, up from $153.6 million in the same period last year.The Nasdaq-listed company parred its losses by 3.55% to $29.9 million during the quarter under review, compared to $31 million in the previous year.
“Freshworks delivered a strong third quarter, with revenue growing 22% year over year to $186.6 million, net cash provided by operating activities margin improving to 23%, and free cash flow margin improving to 21%,” said Dennis Woodside, CEO & President of Freshworks.
“We continue to see mid-market and enterprise companies choose Freshworks as the AI-powered service platform that enables them to scale with exceptional customer and employee experiences,” he added.
Total income from operations amounted to $24 million, up from $17.4 million in the third quarter of 2023.
Earlier in May this year, the California-headquartered firm announced the appointment of Dennis Woodside as the new CEO, with Girish Mathrubootham transitioning to the role of Executive Chairman.
The company reported a rise in its total operating expenses to $195 million in Q3 of 2024, up from $166 million in the corresponding period last year.
The firm reported free cash flow of $40.1 million for the third quarter of 2024, up from $22.1 million a year earlier. As of September 30, 2024, cash, cash equivalents, and marketable securities totaled $1.05 billion.
The net dollar retention rate stood at 107%, compared to 106% in the second quarter of 2024 and 108% in the third quarter of 2023.
Net Dollar Retention (NDR) is a key performance metric that measures how well a company retains and expands revenue from existing customers over time.
The number of customers contributing over $5,000 in annual recurring revenue (ARR) grew 14% year-over-year, reaching 22,359.
In addition, Freshworks onboarded several new customers to its portfolio, including Republic Airways, City of Bellevue, ChampionX, University of Oxford, Sparebank 1, aand TechStyle Fashion Group, among others.
Freshworks also announced that its Board of Directors has approved a stock repurchase programme, authorising the buyback of up to $400 million in outstanding Class A common stock.
A stock repurchase programme or buyback is when a company buys back its own shares from the open market in order to increase the value of the remaining shares by reducing the overall supply.
The firm ended the quarter with the launch of Freddy AI Agent, an easy-to-deploy autonomous service agent designed to enhance both customer experience (CX) and employee experience (EX).
The introduction of Freddy AI Agent comes at a time when many enterprises are adopting AI agents to streamline their workflows. The AI agents can be deployed within minutes and have resolved an average of 45% of customer support requests and 40% of IT service inquiries, said the company in a statement.
In September, Freshworks welcomed former ServiceNow executive Murali Swaminathan as its new Chief Technology Officer. Additionally, after nearly five years, the company’s Chief Product Officer Prakash Ramamurthy stepped down to pursue new career opportunities.
An SEC filing on August 5 announced the appointment of Philippa Lawrence as Chief Accounting Officer. Earlier in the year, Pradeep Rathinam, who served as Chief Revenue Officer, left the company in February.
Startup
Why ‘family’ culture may harm your workplace dynamics
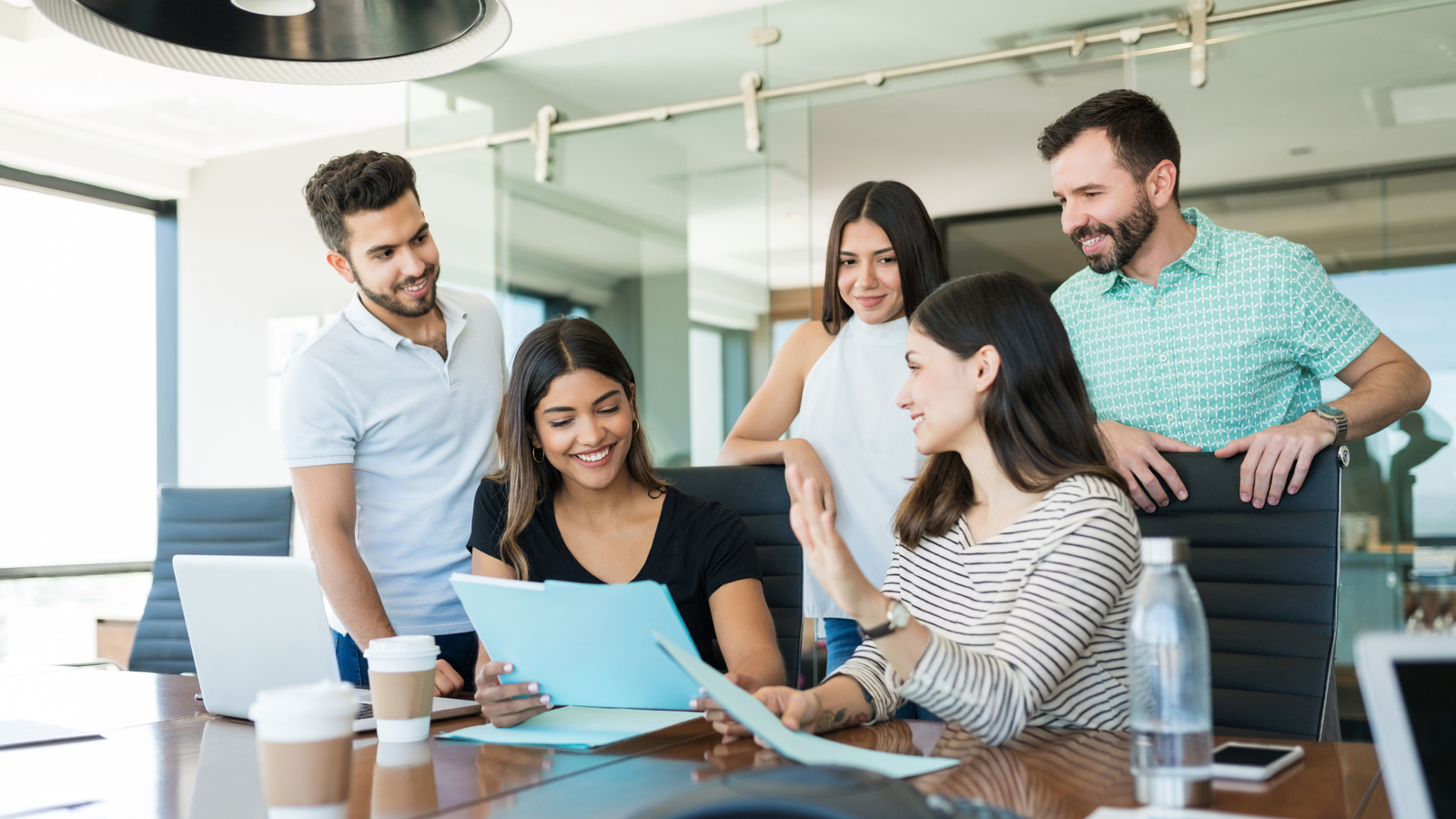
Imagine starting a new job and hearing your manager proudly say, “We’re like a family here.” It sounds warm, inviting, and comforting. But while this sentiment is well-meaning, it may not be the most effective way to frame a business environment. The “family” metaphor is appealing because it promises loyalty, unconditional support, and unity. However, it also implies blurred boundaries, potentially toxic relationships, and unbalanced expectations.
In the realm of business, where clarity, structure, and professionalism are paramount, treating your company as a ‘family’ may set you up for challenges that undermine both productivity and well-being.
The origins of the ‘family’ mentality
The idea of the workplace as a family emerged from a desire to foster camaraderie and trust among employees. Companies wanted to create spaces where people felt safe and motivated, boosting morale and retention. While intentions may have been noble, the execution often leads to problematic outcomes.
Why the ‘family’ mentality falls short
Blurred boundaries and unrealistic expectations
Families are typically associated with unconditional support. However, in a business setting, this mindset can lead to unrealistic expectations where employees feel pressured to go above and beyond their professional scope. This often leads to burnout, as employees may struggle to say no or set boundaries out of fear of disappointing their ‘family.’
Difficulty in addressing underperformance
In a true family, love and loyalty often override consequences for poor behaviour. In a business, however, the stakes are different. Maintaining a ‘family’ mindset can make it difficult for leaders to objectively address underperformance or make tough decisions like layoffs. This approach can create a culture where mediocrity is tolerated, affecting overall productivity and efficiency.
Favouritism and unbalanced treatment
Family-like dynamics can also lead to favouritism or perceptions of unequal treatment, fostering resentment among team members. In a business context, it’s vital to maintain fairness and transparency. The ‘family’ approach may compromise this, as leaders might subconsciously favour those they feel closer to, creating division and tension among teams.
Toxic relationships and emotional burden
Families can have complex, sometimes toxic, dynamics that don’t translate well into professional settings. The ‘like a family’ mantra can encourage employees to bear the emotional weight of their coworkers’ problems or poor behaviour. This added emotional labour is unsustainable and distracts from the primary focus of achieving shared business goals.
Resistance to change
Families tend to value tradition and continuity, sometimes at the expense of adaptability. For a company, clinging to the ‘family’ ideology can hinder innovation and growth. Businesses need to be dynamic, and capable of making strategic shifts without being tied down by the emotional attachments that come with a familial mindset.
What companies should aspire to instead?
Instead of aiming to be ‘like a family,’ companies should adopt a framework that prioritises clear roles, responsibilities, and support structures that respect personal and professional boundaries. Here’s what companies can aim for:
- A supportive community: Unlike a family, a community is built on mutual interests and goals, with a shared sense of purpose but clear boundaries.
- A high-performing team: Teams are focused on collaboration and mutual accountability, maintaining professionalism while fostering trust.
- An empowering culture: Cultivate an environment that encourages personal growth and collective success without imposing unrealistic emotional burdens.
While it’s tempting for companies to aspire to be ‘like a family’ for the sake of unity and morale, it often comes at the cost of professionalism, clear boundaries, and effective leadership. Instead, organisations should strive for a balanced culture that upholds mutual respect, accountability, and a shared vision. The key to long-term success lies in creating a work environment that empowers individuals while maintaining clear lines between the personal and the professional.
Startup
Inflexor Ventures raises Rs 280 Cr as first close for Opportunities Fund
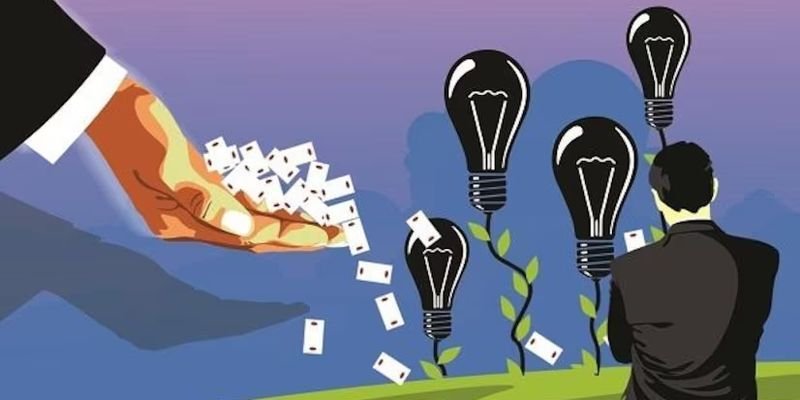
Early-stage venture capital firm Inflexor Ventures raised Rs 280 crore as the first close of its Rs 350 crore Opportunities Fund, raising capital from a diverse set of investors.
In a statement, Inflexor said that HDFC AMC Select AIF FoF I Scheme is the dominant limited partner in the fund. Besides, it also saw participation from HNIs, family offices, corporates, and other institutions.
The VC firm aims to achieve the final close of the fund by the end of this month.
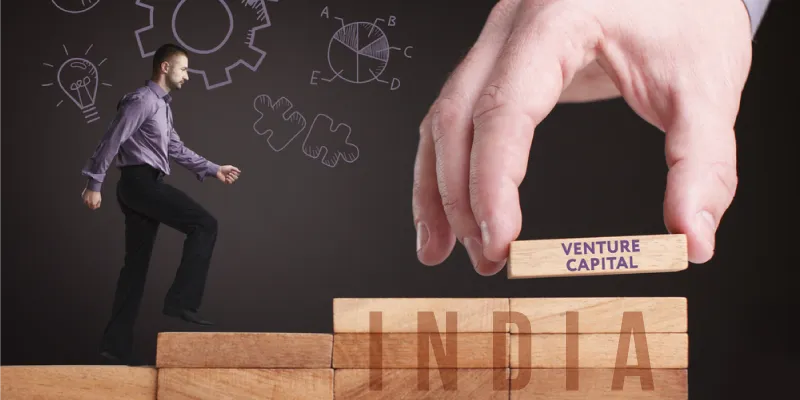
Founded by Venkat Vallabhaneni, Jatin Desai, and Pratip Mazumdar, Inflexor Ventures is a sector-agnostic VC firm managing Rs 1,000 crore of assets under management (AUM) with a portfolio of 26 startups.
According to the VC firm, this transaction has generated returns and liquidity for all its existing Fund-I investors, including IDFC Limited and Sumankant Munjal Family Office through a full portfolio sale of assets.
The VC firm noted that part of the target corpus of the Opportunities Fund will be allocated over the next three to five years to maintain or increase stake in their portfolio companies.
“The first close within six months, bolstered by a sizeable investment from HDFC AMC, underscores strong investor confidence in the underlying portfolio,” said Pratip Mazumdar, Partner at Inflexor.
Inflexor is currently deploying from its second fund raised during 2021 and has invested in companies, including Kale Logistics, Atomberg, PlayShifu, ClickPost, and BioPrime.
It also sold stakes and exited one of its second fund’s portfolio companies Steradian Semiconductors to a Tokyo-listed strategic Japanese company.
Inflexor has been an early investor in startups like Atomberg, Kale Logistics, Entropik, Bellatrix, Clickpost, and Cloudsek.
-
Startup Stories1 year ago
Why Millennials, GenZs Are Riding The Investment Tech Wave In India
-
Startup Stories1 year ago
Startups That Caught Our Eyes In September 2023
-
Startup Stories1 year ago
How Raaho Is Using Tech To Transform India’s Fragmented Commercial Trucking
-
Startup Stories12 months ago
Meet The 10 Indian Startup Gems In The Indian Jewellery Industry’s Crown
-
Crptocurrency8 months ago
Lither is Making Crypto Safe, Fun, and Profitable for Everyone!
-
Startup Stories1 year ago
How Volt Money Is Unlocking The Value Of Mutual Funds With Secured Lending
-
Startup Stories1 year ago
Why Moscow-Based Kladana Considers Indian SME Sector As The Next Big Market For Cloud Computing
-
E-commerce1 year ago
Top Online Couponing Trends To Watch Out For In 2016