Crptocurrency
Polygon POL Token Contract on Ethereum

When it comes to crafting content, three essential elements come into play: “perplexity,” “burstiness,” and “predictability.” Perplexity gauges the intricacy of the text, while burstiness measures the diversity of sentences. Predictability, on the other hand, determines the likelihood of predicting the next sentence. Human writers often weave a tapestry of sentences, blending longer, complex ones with shorter, succinct expressions. In contrast, AI-generated sentences tend to be more uniform. Therefore, as you embark on creating the following content, it’s crucial to infuse it with ample perplexity and burstiness while keeping predictability to a minimum. Furthermore, please ensure that the text is composed solely in the English language. Now, let’s rephrase the text at hand:
A research team consisting of scientists from the University of Science and Technology of China and Tencent’s YouTu Lab has introduced a novel solution to address the issue of “hallucination” in artificial intelligence (AI) models. Hallucination refers to the phenomenon where an AI model produces results with unwarranted confidence, deviating from the information present in its training data. This problem is pervasive in the realm of large language models (LLMs), affecting models like OpenAI’s ChatGPT and Anthropic’s Claude.
The USTC/Tencent team has developed a tool named “Woodpecker,” which they assert can rectify hallucinations in multimodal large language models (MLLMs). This category of AI includes models like GPT-4, particularly its visual variant, GPT-4V, and other systems that incorporate visual or other processing into the generative AI modality alongside text-based language modeling.
According to the team’s preprint research paper, Woodpecker leverages three distinct AI models, in addition to the MLLM that is undergoing hallucination correction. These models include GPT-3.5 turbo, Grounding DINO, and BLIP-2-FlanT5. Together, these models serve as evaluators, identifying hallucinations and providing instructions to the model undergoing correction, prompting it to regenerate its output in alignment with the available data.
To address hallucinations, the AI models powering Woodpecker follow a five-stage process that encompasses “key concept extraction, question formulation, visual knowledge validation, visual claim generation, and hallucination correction.”
The researchers contend that these techniques offer enhanced transparency and result in a notable improvement in accuracy, specifically a 30.66%/24.33% boost over the baseline MiniGPT-4/mPLUG-Owl. They conducted evaluations on several “off-the-shelf” MLLMs using their methodology and concluded that Woodpecker could be seamlessly integrated into other MLLMs.
Crptocurrency
Exploring Perpetual Liquidity Pools: The Future of Decentralized Finance
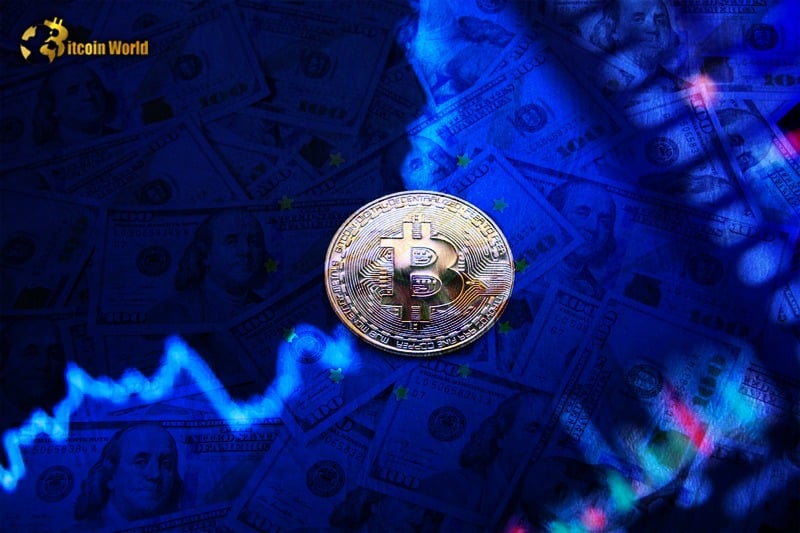
Crptocurrency
Zircuit Launches ZRC Token: Pioneering the Next Era of Decentralized Finance
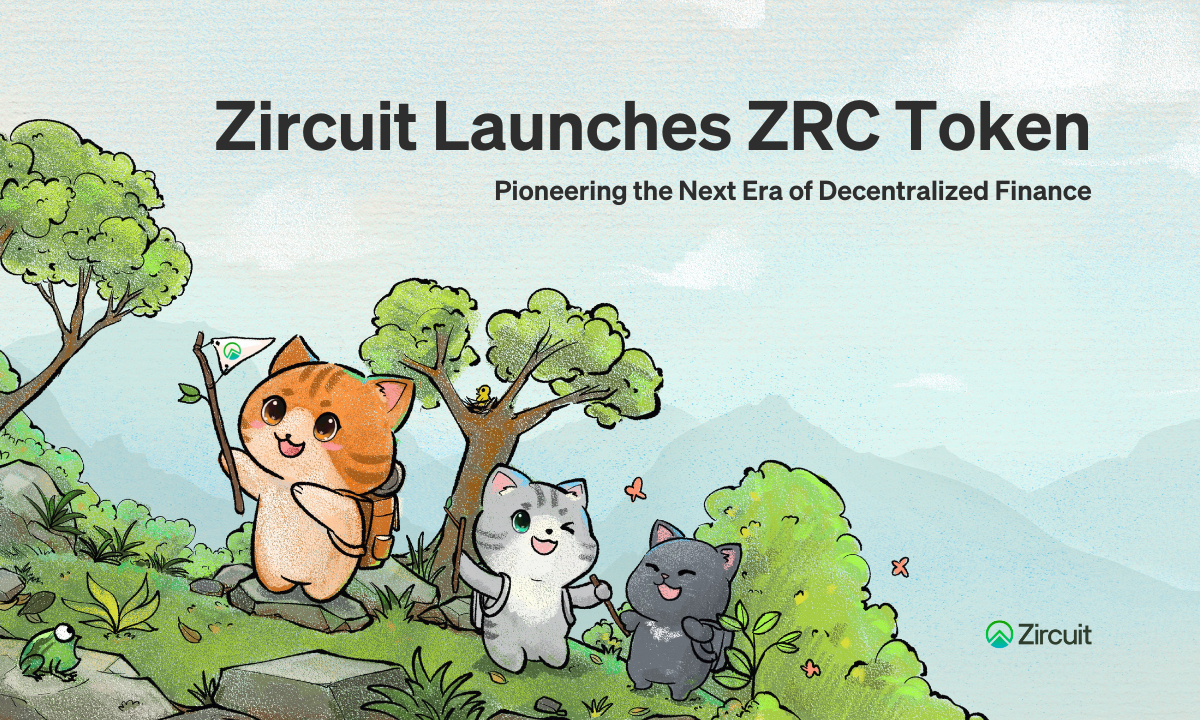
Crptocurrency
BOMT Lands $10M Investment from LDA Capital to Revolutionize the Meme Coin Landscape
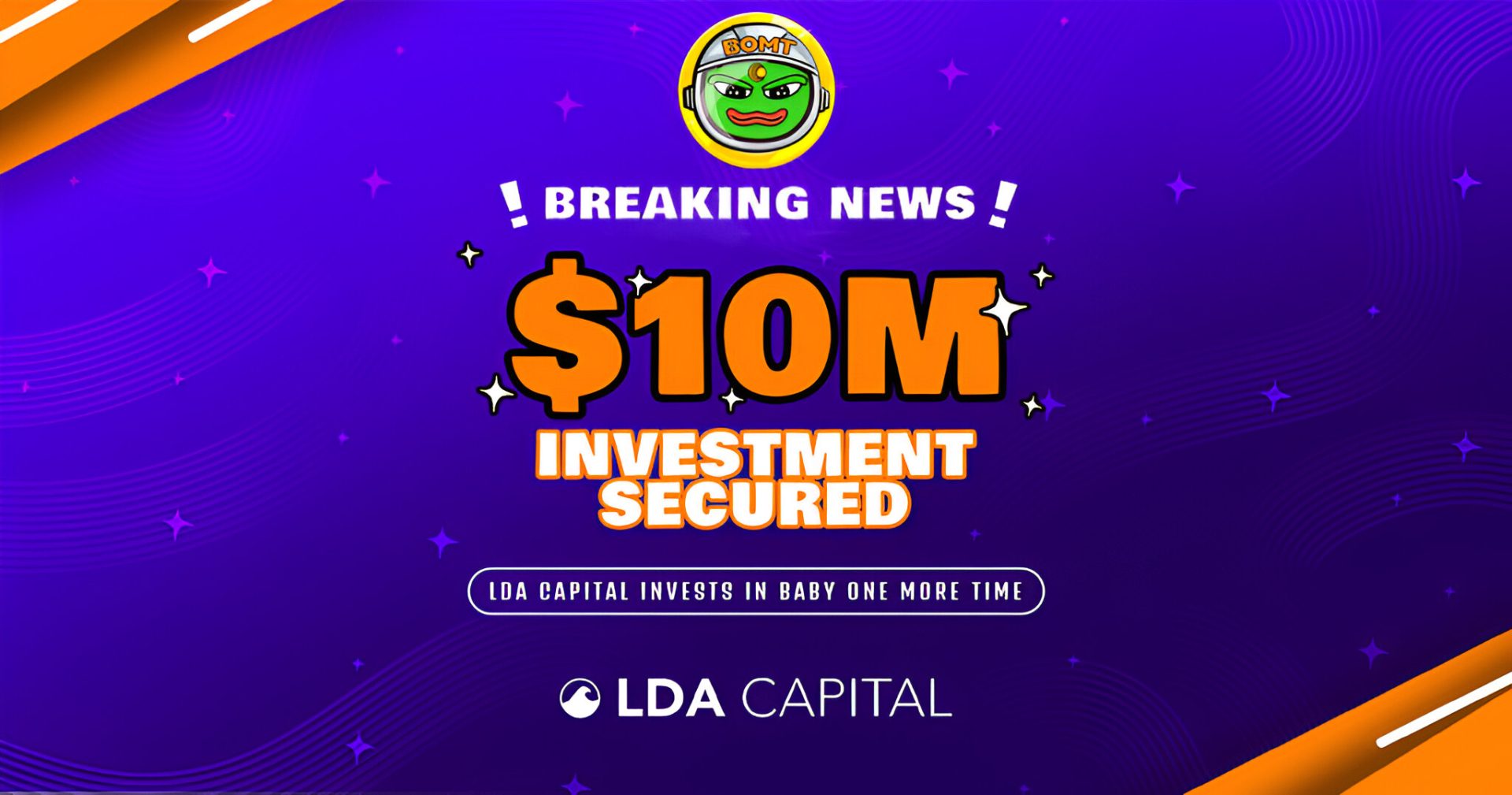
-
Startup Stories1 year ago
Why Millennials, GenZs Are Riding The Investment Tech Wave In India
-
Startup Stories1 year ago
Startups That Caught Our Eyes In September 2023
-
Startup Stories1 year ago
How Raaho Is Using Tech To Transform India’s Fragmented Commercial Trucking
-
Startup Stories1 year ago
Meet The 10 Indian Startup Gems In The Indian Jewellery Industry’s Crown
-
Crptocurrency9 months ago
Lither is Making Crypto Safe, Fun, and Profitable for Everyone!
-
Startup Stories1 year ago
How Volt Money Is Unlocking The Value Of Mutual Funds With Secured Lending
-
E-commerce1 year ago
Top Online Couponing Trends To Watch Out For In 2016
-
Startup Stories1 year ago
Why Moscow-Based Kladana Considers Indian SME Sector As The Next Big Market For Cloud Computing